I haven’t blogged in a while about the Stu build system. Stu is a piece of software written by myself at the University of Namur that we use to run the calculations of network statistics in the KONECT project, build Latex documents, and do all other sorts of data manipulation, and more. The Stu build system is a domain-specific programming language used to build things. This is similar to the well-know Make system, but can do much more. In the KONECT project, we use it in ways that are impossible with Make. In this post, I will show a very short snippet of a Stu script, as it is used in KONECT, and will explain what it does line by line.
Here is the snippet:
#
# Diadens
#
@diadens: @diadens.[dat/NETWORKS_TIME];
@diadens.$network: plot/diadens.a.$network.eps;
plot/diadens.a.$network.eps:
m/diadens.m
$[ -t MATLABPATH]
dat/stepsi.$network
dat/statistic_time.full.(diameter avgdegree).$network
{
./matlab m/diadens.m
}
This code is written in Stu, and is used as shown here in KONECT. It is part of the (much larger) file main.stu in the KONECT-Analysis project. I have left in the comments as they appear in the original. In fact, comments in Stu start with #
, and you can probably recognize that the first three lines are a comment:
#
# Diadens
#
The name “Diadens” refers to diameter-density; we’ll explain later what that means.
The comment syntax in Stu is the same as in many other scripting languages such as the Shell, Perl, Python, etc. Until now, there’s nothing special. Let’s continue:
@diadens: @diadens.[dat/NETWORKS_TIME];
Now we’re talking. In order to explain this line, I must first explain the overall structure of a Stu script: A Stu script consists of individual rules. This one line represents a rule in itself. Of course, rules may be much longer (as we will see later), but this rule is just a single line. As a general rule, Stu does not care about whitespace (up to a few cases which we’ll see later). This means that lines can be split anywhere, and a rule can consist of anything from a single to many hundreds of lines, even though in practice almost all rules are not longer than a dozen lines or so.
The overall way in which Stu works is similar to that of Make. If you know Make, the following explanation will appear trivial to you. But if you don’t, the explanation is not complex either: Each rule tells Stu how to build one or more things. Rules are the basic building blocks of a Stu script, just likes functions are the basic building blocks of a program in C, or classes are the basic building blocks in Java. The order of rules in a Stu script does not matter. This is similar to how in C, it does not matter in which order functions are given, up to the detail that in C, functions have to be declared before they are used. In Stu, there is no separate “declaration” of rules, and therefore the ordering is truly irrelevant. The only exception to this rule is which rule is given first in a complete Stu script, which will determine what Stu does by default, like the main() function in C. This is however not relevant to our snippet, as it is taken from the middle of the Stu script. Let us look as the last rule definition again:
@diadens: @diadens.[dat/NETWORKS_TIME];
Here, the first @diadens
is the target of the rule, i.e., it declares what this rule is able to build. Most rules usually build a file, and on can just write a filename as a target, which means that the goal of the rule is to build the given file. In this case however, the target begins with @
and that means that the target is a so-called transient target. A transient target is just Stu’s way of saying that no file is built by the rule, but we still need to do something to build the rule. In this case, everything after the colon :
are the dependencies of the rule. The meaning is the following: In order to build the target @diadens
, Stu must first build @diadens.[dat/NETWORKS_TIME]
. The semicolon ;
then indicates the end of the rule. Instead of a semicolon, it is also possible to specificy a command that Stu must executes, by enclosing it in braces { }
. In this rule, there is no command, and therefore a semicolon is used.
At this point, I should give a short explanation about was this example is supposed to do: the name diadens
stands for “diameter and density”. The goal of this code is to draw what we may call “diameter and density” plots. These are plots drawn by KONECT which show, for one given dataset of KONECT, the values of the network diameter (a measure of how many “steps” nodes are apart) and the values of the network density (a measure of how many neighbors nodes typically have), as a function of time. The details of the particular calculation do not matter, but for the curious, the goal of this is to verify empirically whether in real-world networks, diameters are shrinking while the density is increasing. Suffice it to say that for each datasets in the KONECT project, we want to generate a plot that looks like this:
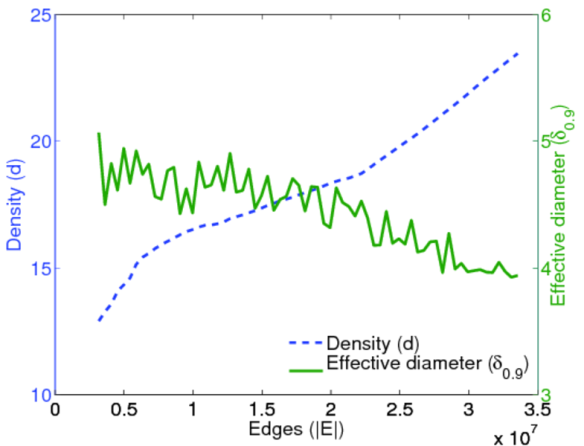
This particular plot was generated for the Wikipedia growth network dataset. As predicted, the diameter (green curve) is shrinking, while the density (purple curve) is increasing. Regardless of the actual behavior of each dataset, we would like to generate these plots for all datasets in the KONECT project. However, there are complications: (1) If we just write a loop over all networks to generate all plots, this will take way too much time. Instead we want to only generate those plots that have not yet been generated. (2) We must restrict the plotting to only those datasets that are temporal, i.e. for datasets that don’t include timestamps, we cannot generate this plot. The Stu snippet takes care of both aspects. Let’s now look at the dependency:
@diadens.[dat/NETWORKS_TIME]
As always in Stu, if a name starts with a @
, it is a transient. In this case however, it is a little bit more complex than what we have seen before. In this case, brackets [ ]
are used. What is the purpose of the brackets in Stu? They indicate that part of the name is to be generated dynamically. In this case, it means that Stu must first build dat/NETWORKS_TIME
(which is a file because it does not start with @
), and then the brackets [dat/NETWORKS_TIME]
will be replaced by the content of the file dat/NETWORKS_TIME
. The file dat/NETWORKS_TIME
is a file of KONECT which contains the name of all datasets which have timestamps, all names being separated by whitespace. This is exactly the list of datasets to which we want to apply our calculation. In fact, the main.stu
file of KONECT also contains a rule for building the file dat/NETWORKS_TIME
, which has to be regenerated whenever new datasets are added to the project. Now, we said that Stu will replace the string [dat/NETWORKS_TIME]
with the content of the file dat/NETWORKS_TIME
— this is not 100% precise. Since that file contains multiple names (one for each dataset), Stu will duplicate the whole dependency @diadens.[dat/NETWORKS_TIME]
, once for each name in the file dat/NETWORKS_TIME
. As a result, the dependencies of @diadens
will be of the form @diadens.XXX
, where XXX
is replaced with each dataset of KONECT that has timestamps. This exactly what we want: We have just told Stu that in order to build @diadens
(i.e., build all diameter-density plots), Stu must build all @diadens.XXX
plots (i.e., each individual diameter-density plot), for each dataset with timestamps individually. Then, what is the difference between that one line of Stu script, and a loop over all temporal datasets, generating the plots for all datasets in turn? For one, Stu is able to parallelize all work, i.e., it will be able to generate multiple plots in parallel, and in fact the number of things to execute in parallel is determined by the -j
options to Stu (which should be familiar to users of GNU or BSD Make). Second, Stu will not always rebuild all plots, but only those that need to be rebuilt. What this means is that when Stu is invoked as
$ stu @diadens
on the command line, Stu will go through all temporal datasets, and will verify whether: (1) the plot has already been done, and (2) if is has already been done, whether the plotting code has changed since. Only then will Stu regenerate a plot. Furthermore, because the file dat/NETWORKS_TIME
itself has a rule for it in the Stu script, Stu will automatically check whether any new datasets have been added to the KONECT project (not a rare occasion), and will generate all diameter-density plots for those. (Users of Make will now recognize that this is difficult to achieve in Make — most makefiles would autogenerate code to achieve this.)
Now, let us continue in the script:
@diadens.$network: plot/diadens.a.$network.eps;
Again, this is a rule that spans a single line, and since it ends in a semicolon, doesn’t have a command. The target of this rule is @diadens.$network
, a transient. What’s more, this target contains a dollar sign $
, which indicates a parameter. In this case, $network
is a parameter. This means that this rule can apply to more than one target. In fact, this rule will apply to any target of the form @diadens.*
, where *
can be any string. By using the $
syntax, we automatically give a name to the parameter. In this case, the parameter is called $network
, and it can be used in any dependency in that rule. Here, there is just a single dependency: plot/diadens.a.$network.eps
. Its name does not start with @
and therefore it refers to a file. The filename however contains the parameter $network
, which will be replaced by Stu by whatever string was matched by the target of the rule. For instance, if we tell Stu to build the target @diadens.enron
(to build the diameter-density plot of the Enron email dataset), then the actual dependency will be plot/diadens.a.enron.eps
. This is exactly the filename of the image that will contain the diameter-density plot for the Enron email dataset, and of course diameter-density plots in KONECT are stored in files named plot/diadens.a.XXX.eps
, where XXX
is the name of the dataset.
Finally, we come to the last part of the Stu script snippet, which contains code to actually generate a plot:
plot/diadens.a.$network.eps:
m/diadens.m
$[ -t MATLABPATH]
dat/stepsi.$network
dat/statistic_time.full.(diameter avgdegree).$network
{
./matlab m/diadens.m
}
This is a single rule in Stu syntax, which we can now explain line by line. Let’s start with the target:
plot/diadens.a.$network.eps:
This tells Stu that the rule is for building the file plot/diadens.a.$network.eps
. As we have already seen, the target of a rule may contain a parameter such as $network
, in which case Stu will perform pattern-matching and apply the given rule to all files (or transients) which match the pattern. Let’s continue:
m/diadens.m
This is our first dependency. It is a file (because it does not start with @
), and it does not contain the parameter $network
. Thus, whatever the value of $network
is, building the file plot/diadens.a.$network.eps
will always need the file m/diadens.m
to be up to date. This makes sense, of course, because m/diadens.m
is the Matlab code that we use to generate the diameter-density plots. Note that a dependency may (but does not need to) include any parameter used in the target name, but it is an error if a dependency includes a parameter that is not part of the target name.
The next line is $[ -t MATLABPATH]
, but in defiance of linearity of exposition, we will explain that line last, because it is a little bit more complex. It is used to configure the Matlab path, and uses the advanced -t
flag (which stands for trivial). The next line is:
dat/stepsi.$network
This is a file dependency, and, as should be familiar by now, it is parametrized. In other words, in order to build the file plot/diadens.a.$network.eps
, the file dat/stepsi.$network
must be built first. In KONECT, the file dat/stepsi.$network
contains the individual “time steps” for any temporal analysis, i.e., it tells KONECT how many time steps we plot for each temporal dataset, and where the individual “time steps” are. This is because, obviously, we don’t want to recompute measures such as the diameter for all possible time points, but only for about a hundred of them, which is largely enough to create good-looking plots; more detail in the plots would not make them look much different. Unnecessary to mention then, that the Stu script of KONECT also contains a rule for building the file dat/stepsi.$network
. Then, we continue for one more target of this rule:
dat/statistic_time.full.(diameter avgdegree).$network
As before, this target includes the parameter $network
, which will be replaced by the name of the dataset. Furthermore, this line contains a Stu operator we have not yet seen: the parentheses ( )
. The parentheses ( )
in Stu work similarly to the braces [ ]
, but instead of reading content from a file, they take their content directly from what is written between them. Thus, this one line is equivalent to the following two lines:
dat/statistic_time.full.diameter.$network
dat/statistic_time.full.avgdegree.$network
Thus, we see that we are simply telling Stu that the script m/diadens.m
will also access these two files, which simply contain the actual values of the diameter and density over time for the given network. (Note that the density is called avgdegree
internally in KONECT.)
Finally, our snippet ends with
{
./matlab m/diadens.m
}
This is an expression in braces { }
and in Stu, these represent a command. A command can be specified instead of a semicolon when something has actually to be executed for the rule. In most cases, rules for files have commands, and rules for transients don’t. The actual command is not written in Stu, but is a shell script. Stu will simply invoke /bin/sh
to execute it. In this example, we simply execute the Matlab script m/diadens.m
. Here ./matlab
is a wrapper script for executing Matlab scripts, which we use because pure Matlab is not a good citizen when it comes to being called from a shell script. For instance, Stu relies on the exit status (i.e., the numerical exit code) of programs to detect errors. Unfortunately, Matlab always returns the exit status zero (indicating success) even when it fails, and hence we use a wrapper script.
One question you should now be asking is: How does that Matlab script know which plot to generate, let alone which input files (like dat/statistic_time.full.diameter.$network
) to read? The answer is that Stu will pass the value of the parameter $network
as … an environment variable called $network
. Thus, the Matlab code simply uses getenv('network')
, which is the Matlab code to read the environment variable named $network
. We thus see that most commands in Stu script don’t need to use their parameters: these are passed transparently by the shell to any called program.
Finally, we can come back to that one line we avoided earlier:
$[ -t MATLABPATH]
The characters $[
indicate that this is a variable dependency. This means that Stu will first make sure that the file MATLABPATH
is up to date, and then pass the content of that file to the command, as an environment variable of the same name. Therefore, such a variable dependency is only useful when there is a command. In this case, the environment variable $MATLABPATH
is used by Matlab to determine the path in order to find its libraries. The file MATLABPATH
, which contains that path as used in KONECT, has its own rule in the KONECT Stu script, because its content is non-trivial. Had we written $[MATLABPATH]
as a dependency, that would be the end of the story. But that would have a huge drawback: Every time we change the Matlab path, Stu would rebuild everything in KONECT, or at least everything that is built with Matlab, simply because MATLABPATH
is declared as a dependency. Instead, we could have omitted the dependency $[MATLABPATH]
altogether, and simply have our script ./matlab
read out the file MATLABPATH
and set the environment variable $MATLABPATH
accordingly. But that would also not have been good, because then the file MATLABPATH
would never have been built to begin with, when we start with a fresh installation of KONECT. We could also put the code to determine the Matlab path directly into ./matlab
to avoid the problem, but that would mean the starting of every Matlab script would be very slow, as it would have to be generate again each time. We could made ./matlab
cache the results (maybe even in the file MATLABPATH
), but since ./matlab
is called multiple times in parallel, it would have meant that ./matlab
would need some form of a locking mechanism to avoid race conditions. Instead of all that, the -t
flag in Stu does exactly what we want here. -t
stands for trivial, and is used in Stu to mark a dependency as a trivial dependency. This means that the a change in the file MATLABPATH
will not result in everything else being rebuilt. However, if the file MATLABPATH
does not exist, or if it exists but needs to be rebuilt and Stu determines that the command using Matlab must be executed for another reason, then (and only then) does Stu rebuild MATLABPATH
. (For those who know the Make build system: This is one of the features of Stu that are nearly impossible to recreate in Make.) Note that there are also flags in Stu that can be used in a similar way: -p
declares a dependency as persistent (meaning it will never be rebuilt if already present), and -o
as optional (meaning it is only rebuilt, if at all, if the target file is already present).
This concludes our little tour of a little Stu script snippet. Fore more information about Stu, you may read these previous blog articles:
You can get Stu at Github:
For reference, the example in this blog article uses features introduced in Stu version 2.5. Stu is always backward compatible with previous versions, but if you have a pre-2.5 version installed, then of course these new features will not work.
The words written in italics in this article are official Stu nomenclature.
EDIT 2018-01-29: fixed a typo